Central Limit Theorem Sample Mean Calculator
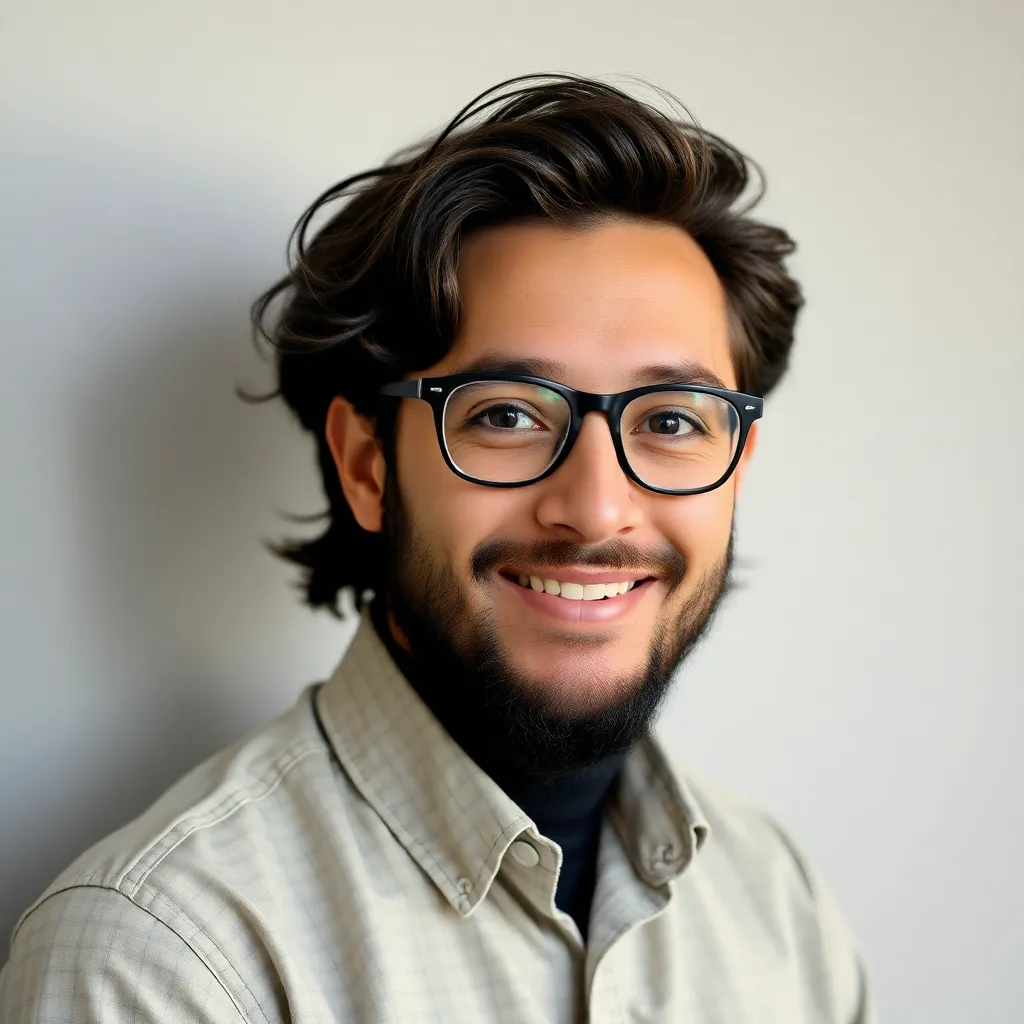
Webtuts
May 10, 2025 · 7 min read
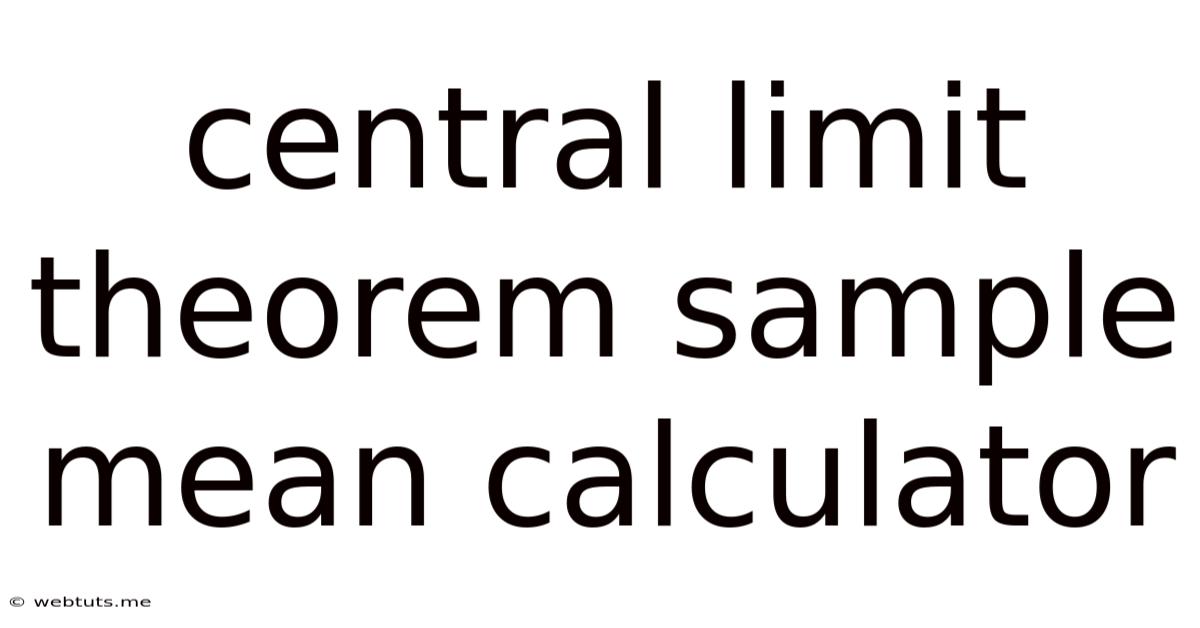
Table of Contents
Central Limit Theorem Sample Mean Calculator: A Deep Dive
The Central Limit Theorem (CLT) is a cornerstone of statistical inference. It states that the distribution of the sample means approximates a normal distribution as the sample size gets larger, regardless of the shape of the population distribution. This powerful theorem allows us to make inferences about a population based on sample data, even if we don't know the population's true distribution. Understanding the CLT and utilizing tools to calculate sample means efficiently are crucial skills for anyone working with statistical data. This article will delve into the intricacies of the Central Limit Theorem, explaining its implications and providing practical guidance on using a sample mean calculator.
Understanding the Central Limit Theorem
The CLT asserts that the distribution of the sample means approaches a normal distribution as the sample size (n) increases, with a mean equal to the population mean (μ) and a standard deviation equal to the population standard deviation (σ) divided by the square root of the sample size (σ/√n). This standard deviation of the sampling distribution is also known as the standard error.
Key aspects of the CLT:
-
Sample Size: The larger the sample size, the closer the sampling distribution of the means will resemble a normal distribution. Generally, a sample size of 30 or more is considered sufficient for the CLT to hold, especially for populations that are not drastically skewed. However, for highly skewed populations, a larger sample size might be necessary.
-
Population Distribution: The CLT applies regardless of the shape of the population distribution. This is its remarkable strength. Whether the population is normally distributed, uniformly distributed, exponentially distributed, or follows any other distribution, the distribution of sample means will tend toward normality as the sample size grows.
-
Independence: The samples must be independent. This means that the selection of one sample point does not influence the selection of another.
-
Standard Error: The standard error (σ/√n) quantifies the variability of the sample means. A smaller standard error indicates that the sample means are clustered more tightly around the population mean, implying greater precision in estimating the population mean.
Practical Implications of the CLT
The CLT has profound implications for statistical inference:
-
Confidence Intervals: The CLT forms the basis for constructing confidence intervals. A confidence interval provides a range of values within which the true population mean is likely to lie, with a specified level of confidence (e.g., 95%). The calculation of confidence intervals heavily relies on the normality of the sampling distribution, which the CLT guarantees for sufficiently large sample sizes.
-
Hypothesis Testing: Many hypothesis tests, such as t-tests and z-tests, rely on the assumption of normality. The CLT justifies this assumption when dealing with sample means, even if the underlying population is not normally distributed. These tests allow us to make inferences about population parameters based on sample data.
-
Sampling Distributions: Understanding sampling distributions is crucial for interpreting statistical results. The CLT helps us predict the behavior of these distributions, making it easier to assess the reliability and validity of our findings.
Using a Central Limit Theorem Sample Mean Calculator
A sample mean calculator simplifies the process of calculating the sample mean and related statistics. While you can manually calculate these values using basic statistical formulas, a calculator significantly reduces the time and effort involved, especially when dealing with large datasets.
Features of a Typical Sample Mean Calculator:
-
Data Input: Most calculators allow you to input data in various formats, such as manually entering individual data points or uploading a data file (CSV, Excel).
-
Sample Mean (x̄): This is the average of the sample data points. The calculator computes this automatically.
-
Sample Standard Deviation (s): This measures the dispersion or spread of the data points around the sample mean.
-
Sample Size (n): The number of data points in the sample.
-
Standard Error (SE): This is the standard deviation of the sampling distribution of the means (s/√n).
-
Confidence Interval: The calculator can compute confidence intervals at various confidence levels (e.g., 90%, 95%, 99%).
-
Visualization: Some advanced calculators provide graphical representations of the data, such as histograms or box plots, to help visualize the data distribution.
Step-by-Step Guide to Using a Sample Mean Calculator (Hypothetical Example)
Let's assume we have the following data representing the weights (in kilograms) of 10 randomly selected pumpkins:
2.5, 3.1, 2.8, 3.5, 3.0, 2.7, 3.2, 2.9, 3.3, 3.4
-
Input the Data: Enter the data points into the sample mean calculator.
-
Calculate the Sample Mean (x̄): The calculator will automatically compute the sample mean, which is the sum of the data points divided by the number of data points. In this example, x̄ ≈ 3.04 kg.
-
Calculate the Sample Standard Deviation (s): The calculator will compute the sample standard deviation, which measures the dispersion of the data around the sample mean. This will be a value representing the variability in pumpkin weights.
-
Calculate the Standard Error (SE): The calculator will compute the standard error (s/√n), which represents the standard deviation of the sampling distribution of the means. This quantifies the precision of our sample mean as an estimate of the population mean.
-
Calculate the Confidence Interval: Specify a confidence level (e.g., 95%). The calculator will then compute the confidence interval, giving you a range of values within which the true population mean of pumpkin weights is likely to lie with 95% confidence.
-
Interpret the Results: Analyze the results to draw conclusions about the population mean. For example, a 95% confidence interval of (2.8, 3.3) would suggest that we are 95% confident that the true population mean pumpkin weight lies between 2.8 and 3.3 kg.
Advanced Applications and Considerations
The CLT's applications extend far beyond simple sample mean calculations. Here are some more advanced considerations:
-
Non-Normal Populations: Even with non-normal populations, the CLT still provides valuable approximations for large sample sizes. However, for smaller sample sizes from highly skewed populations, using non-parametric methods might be more appropriate.
-
Sampling with Replacement vs. Without Replacement: The CLT assumes sampling with replacement. If sampling is done without replacement from a finite population, the standard error calculation needs to be adjusted using a finite population correction factor.
-
Central Limit Theorem for Proportions: The CLT can also be applied to sample proportions. The sampling distribution of sample proportions will approximate a normal distribution for large sample sizes, with a mean equal to the population proportion and a standard deviation equal to √(p(1-p)/n), where p is the population proportion and n is the sample size.
-
Simulation: The CLT can be demonstrated visually through simulations. By repeatedly sampling from a population and plotting the distribution of sample means, one can observe the convergence towards a normal distribution as the sample size increases. This is a powerful way to build intuition about the theorem.
Importance of Sample Size
The sample size is a crucial factor influencing the accuracy of estimates derived from the CLT. Larger sample sizes generally lead to:
-
Smaller Standard Error: Reducing the uncertainty in the estimation of the population mean.
-
More Accurate Confidence Intervals: Narrower confidence intervals, suggesting a more precise estimate of the population mean.
-
Better Approximation to Normality: The sampling distribution of means converges more closely to a normal distribution.
However, it's important to note that excessively large sample sizes aren't always necessary or practical. The optimal sample size depends on factors such as the desired level of precision, the variability in the population, and the resources available for data collection.
Conclusion
The Central Limit Theorem is a fundamental concept in statistics, providing a powerful framework for making inferences about population means based on sample data. Understanding the CLT and effectively using sample mean calculators are essential skills for anyone working with statistical data. The CLT's broad applicability, combined with the ease of use provided by readily available sample mean calculators, makes it an indispensable tool for researchers and analysts across various fields. While manual calculations can be performed, utilizing a calculator streamlines the process, allowing for more efficient analysis and interpretation of results. By mastering these concepts, you can confidently leverage the power of the CLT to gain valuable insights from your data. Remember to always consider the assumptions and limitations of the CLT when applying it in your analysis.
Latest Posts
Latest Posts
-
How Many Pounds Is 23 Ounces
May 11, 2025
-
How Many Days Until December 16th 2024
May 11, 2025
-
How Many Inches Is 1 8 Of A Yard
May 11, 2025
-
How Much Sugar Is 38 Grams
May 11, 2025
-
How Many Days Ago Was November 11
May 11, 2025
Related Post
Thank you for visiting our website which covers about Central Limit Theorem Sample Mean Calculator . We hope the information provided has been useful to you. Feel free to contact us if you have any questions or need further assistance. See you next time and don't miss to bookmark.